PINV Moore-Penrose Pseudoinverse
Section: Array Generation and Manipulations
Usage
Calculates the Moore-Penrose pseudoinverse of a matrix. The general syntax for its use isy = pinv(A,tol)
or for a default specification of the tolerance tol
,
y = pinv(A)
For any m x n
matrix A
, the Moore-Penrose pseudoinverse
is the unique n x m
matrix B
that satisfies the following
four conditions
-
A B A = A
-
B A B = B
-
(A B)' = A B
-
(B A)' = B A
B y
is the minimum norm, least squares
solution to A x = y
. The Moore-Penrose pseudoinverse is computed
from the singular value decomposition of A
, with singular values
smaller than tol
being treated as zeros. If tol
is not specified
then it is chosen as
tol = max(size(A)) * norm(A) * teps(A).
Function Internals
The calculation of the MP pseudo-inverse is almost trivial once the svd of the matrix is available. First, for a real, diagonal matrix with positive entries, the pseudo-inverse is simply
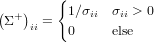
One can quickly verify that this choice of matrix satisfies the
four properties of the pseudoinverse. Then, the pseudoinverse
of a general matrix A = U S V'
is defined as

and again, using the facts that U' U = I
and V V' = I
, one
can quickly verify that this choice of pseudoinverse satisfies the
four defining properties of the MP pseudoinverse. Note that in
practice, the diagonal pseudoinverse S^{+}
is computed with
a threshold (the tol
argument to pinv
) so that singular
values smaller than tol
are treated like zeros.
Examples
Consider a simple1 x 2
matrix example, and note the various
Moore-Penrose conditions:
--> A = float(rand(1,2)) A = 0.9526 0.4847 --> B = pinv(A) B = 0.8338 0.4243 --> A*B*A ans = 0.9526 0.4847 --> B*A*B ans = 0.8338 0.4243 --> A*B ans = 1.0000 --> B*A ans = 0.7943 0.4042 0.4042 0.2057
To demonstrate that pinv
returns the least squares solution,
consider the following very simple case
--> A = float([1;1;1;1]) A = 1 1 1 1
The least squares solution to A x = b
is just x = mean(b)
,
and computing the pinv
of A
demonstrates this
--> pinv(A) ans = 0.2500 0.2500 0.2500 0.2500
Similarly, we can demonstrate the minimum norm solution with the following simple case
--> A = float([1,1]) A = 1 1
The solutions of A x = 5
are those x_1
and x_2
such that
x_1 + x_2 = 5
. The norm of x
is x_1^ + x_2^2
, which is
x_1^2 + (5-x_1)^2
, which is minimized for x_1 = x_2 = 2.5
:
--> pinv(A) * 5.0 ans = 2.5000 2.5000